Bias in machine learning models can have far-reaching and detrimental effects. With the increasing use of AI to automate or support decision-making in a wide variety of fields, there is a pressing need for bias assessment methods that take into account both the statistical detection and the social impact of bias in a context-sensitive way. This is why we present a comprehensive, two-pronged approach to addressing algorithmic bias. Our approach comprises two components: (1) a quantitative bias scan tool, and (2) a qualitative, deliberative assessment. In this way, the scalable and data-driven benefits of machine learning work in tandem with the normative and context-sensitive judgment of human experts, in order to determine fair AI in a concrete way.
Our bias scan tool, which forms the quantitative component, aims to discover complex and hidden forms of bias. The tool is specifically geared towards detecting unforeseen forms of bias and higher-dimensional forms of bias. Aside from unfair biases with respect to established protected groups, such as gender, sexual orientation, and race, bias can also occur with respect to non-established and unexpected groups of people. These forms of bias are more difficult to detect for humans, especially when the unfairly treated group is defined by a high-dimensional mixture of features. Our bias scan tool is based on an unsupervised clustering method, which makes it capable of detecting these complex forms of bias. It thereby tackles the difficult problem of detecting proxy discrimination that stems from unforeseen and higher-dimensional forms of bias, including intersectional forms of discrimination.
Informed by the quantitative results of our bias scan tool, subject-matter experts carry out an evaluative assessment in a deliberative way. The deliberative assessment provides a deeper understanding and evaluation of the detected bias. Such an assessment is essential for a balanced, normative assessment of the bias and its potential consequences in terms of social impact. This is because factual observation of quantitative discrepancies does not establish discrimination or unfair bias by itself. Instead, quantitative discrepancies can only serve as a starting point for evaluating the possibility of unfair treatment in a qualitative way, where the qualitative assessment takes into account the particular social context and the relevant legal doctrines. Human interpretation is thereby required to establish which statistical disparities do indeed qualify as unfair bias. The diversity of our commission of experts contributes to a context-sensitive and multi-perspectival evaluation of the particular use case under investigation. This expert-led, deliberative approach is commonly used by NGO Algorithm Audits to provide ethical guidance on issues that arise in concrete algorithmic use cases. We are our Joint Fairness Assessment Method currently testing at two Dutch public sector organizations. The results of deliberative assessments will be published in a transparent way. We thereby enable policymakers, journalists, data subjects, and other stakeholders to review the normative judgements issued by the audit commissions of Algorithm Audit, thereby contributing to public knowledge building on the responsible use of AI.
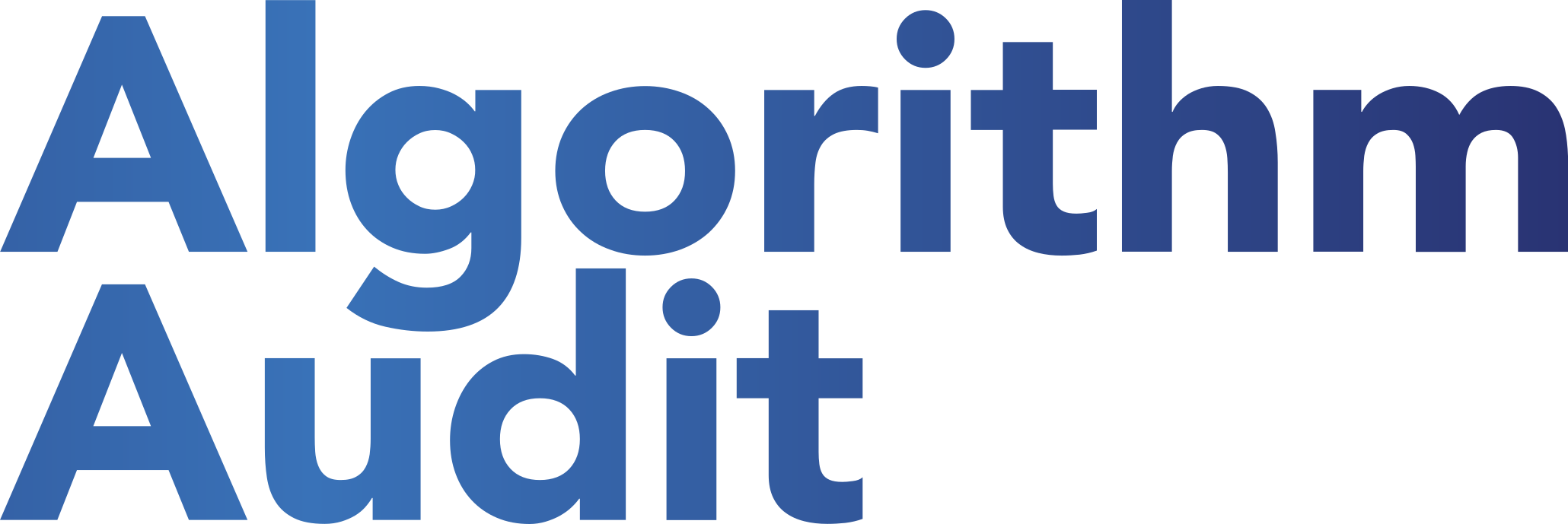

Type
Solution

Organisation
NGO Algorithm Audit

Country
Netherlands